Package: POWSC 1.13.0
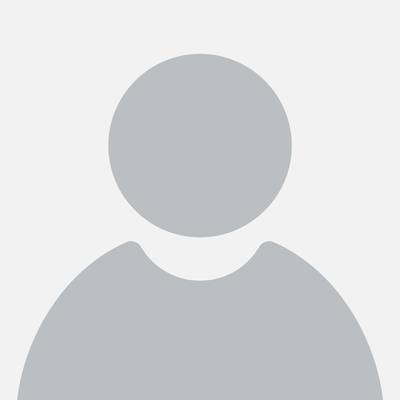
Kenong Su
POWSC: Simulation, power evaluation, and sample size recommendation for single cell RNA-seq
Determining the sample size for adequate power to detect statistical significance is a crucial step at the design stage for high-throughput experiments. Even though a number of methods and tools are available for sample size calculation for microarray and RNA-seq in the context of differential expression (DE), this topic in the field of single-cell RNA sequencing is understudied. Moreover, the unique data characteristics present in scRNA-seq such as sparsity and heterogeneity increase the challenge. We propose POWSC, a simulation-based method, to provide power evaluation and sample size recommendation for single-cell RNA sequencing DE analysis. POWSC consists of a data simulator that creates realistic expression data, and a power assessor that provides a comprehensive evaluation and visualization of the power and sample size relationship.
Authors:
POWSC_1.13.0.tar.gz
POWSC_1.13.0.zip(r-4.5)POWSC_1.13.0.zip(r-4.4)POWSC_1.13.0.zip(r-4.3)
POWSC_1.13.0.tgz(r-4.4-any)POWSC_1.13.0.tgz(r-4.3-any)
POWSC_1.13.0.tar.gz(r-4.5-noble)POWSC_1.13.0.tar.gz(r-4.4-noble)
POWSC_1.13.0.tgz(r-4.4-emscripten)POWSC_1.13.0.tgz(r-4.3-emscripten)
POWSC.pdf |POWSC.html✨
POWSC/json (API)
NEWS
# Install 'POWSC' in R: |
install.packages('POWSC', repos = c('https://bioc.r-universe.dev', 'https://cloud.r-project.org')) |
- es_mef_sce - Sample data for POWSC
- sce - Sample data for GSE67835
On BioConductor:POWSC-1.13.0(bioc 3.20)POWSC-1.12.0(bioc 3.19)
This package does not link to any Github/Gitlab/R-forge repository. No issue tracker or development information is available.
Last updated 2 months agofrom:2cefe0c4cb
Exports:Est2Phaseplot_POWSCPower_ContPower_DiscrunDErunPOWSCSimulate2SCESimulateMultiSCEssummary_POWSC
Dependencies:abindaskpassBiobaseBiocGenericsclicolorspacecrayoncurldata.tableDelayedArrayfansifarverGenomeInfoDbGenomeInfoDbDataGenomicRangesggplot2gluegtablehmshttrIRangesisobandjsonlitelabelinglatticelifecyclelimmamagrittrMASSMASTMatrixMatrixGenericsmatrixStatsmgcvmimemunsellnlmeopensslpheatmappillarpkgconfigplyrprettyunitsprogressR6RColorBrewerRcppreshape2rlangS4ArraysS4VectorsscalesSingleCellExperimentSparseArraystatmodstringistringrSummarizedExperimentsystibbleUCSC.utilsutf8vctrsviridisLitewithrXVectorzlibbioc